Places_512_fulldata_g 目录 , Architecture, Maximizing & More
Introduction
The places_512_fulldata_g 目录 has caused a insurgency in the field of picture inpainting, advertising a capable instrument to upgrade and reestablish computerized pictures. This progressed demonstrate, with its places_512_fulldata_g.pth 路径, has demonstrated basic for experts and devotees alike, giving a strong arrangement to fill in lost or harmed parts of pictures with surprising exactness. Its capacity to get it setting and produce reasonable substance has made it a game-changer in different businesses, from photography to advanced restoration.
This direct points to walk perusers through the successful utilize of the places_512_fulldata_g 目录. It will cover the model’s engineering, clarifying how it forms and gets it picture information. Perusers will learn how to get ready pictures for inpainting, guaranteeing ideal comes about. The article will too dive into strategies to maximize execution and quality, making a difference clients get the most out of this effective device. By the conclusion, perusers will have a strong get a handle on of how to utilize places_512_fulldata_g 目录 to its full potential in their projects.
Places_512_fulldata_g Demonstrate Architecture
The places_512_fulldata_g 目录 and its related places_512_fulldata_g.pth 路径 speak to a advanced inpainting demonstrate outlined to upgrade and reestablish advanced pictures with surprising precision. This model’s engineering is built upon the establishment of Steady Dissemination, consolidating particular adjustments to exceed expectations in the errand of picture inpainting.
Technical Overview
The places_512_fulldata_g 目录 demonstrate is based on the Steady Dissemination 1.5 design, which has been fine-tuned for inpainting assignments . This specialized preparing handle includes a two-step approach: to begin with, 595,000 steps of standard preparing, taken after by 440,000 steps of inpainting-specific preparing at a determination of 512×512 pixels . This dual-phase preparing empowers the demonstrate to get it both total pictures and the subtleties of filling in conceal regions.
Key Components and Layers
The center of the places_512_fulldata_g 目录 show is a altered UNet engineering, which is significant for its inpainting capabilities. The UNet in this demonstrate highlights five extra input channels compared to standard era models . Four of these channels are committed to encoding the conceal picture, whereas the fifth channel speaks to the cover itself. This extra input permits the demonstrate to handle both the existing picture substance and the ranges that require to be filled.
The model’s design incorporates a few key layers:
- Input Layer: Acknowledges the unique picture and mask
- Encoding Layers: Prepare and downsample the input
- UNet Center: Performs the primary inpainting computations
- Decoding Layers: Upsample and refine the output
5.Output Layer: Produces the last inpainted image
Comparison with Other Inpainting Models
The places_512_fulldata_g 目录 show offers a few focal points over standard picture era models when it comes to inpainting tasks:
- Contextual Understanding: Not at all like common picture era models, inpainting models like places_512_fulldata_g 目录 are prepared on both full and halfway (conceal) pictures, permitting them to way better get it and keep up the setting of the existing picture .
- Edge Consistency: Inpainting models deliver comes about with less recognizable edges where the cover was connected, making more consistent integrative .
- Prompt Comprehension: When given particular informational, inpainting models tend to have superior provoke comprehension for the regions being filled, coming about in more precise and relevantly fitting augmentations .
- Outpainting Capabilities: Whereas essentially planned for inpainting, these models moreover exceed expectations at outpainting assignments, viably amplifying pictures past their unique boundaries .
Maximizing Execution and Quality
Optimizing GPU Usage
To maximize the execution of the places_512_fulldata_g 目录 and its related places_512_fulldata_g.pth 路径, optimizing GPU utilization is significant. GPUs can essentially quicken profound learning demonstrate preparing due to their specialized tensor operations . Checking measurements such as GPU utilization, memory utilization, and control utilization gives profitable experiences into asset utilization and potential regions for enhancement .
One compelling methodology to improve GPU utilization is mixed-precision preparing. This strategy utilizes distinctive floating-point sorts (e.g., 32-bit and 16-bit) to move forward computing speed and decrease memory utilization whereas keeping up exactness . Mixed-precision preparing permits for bigger bunch sizes, possibly multiplying them, which essentially boosts GPU utilization .
Another approach to optimize GPU utilization includes making strides information exchange and handling. Caching habitually gotten to information and utilizing CPU-pinned memory can encourage speedier information exchange from CPU to GPU memory . Moreover, NVIDIA’s Information Stacking Library (DALI) can be utilized to construct highly-optimized information preprocessing pipelines, offloading particular assignments to GPUs .
Balancing Speed and Yield Quality
Achieving a adjust between speed and yield quality is basic when working with the places_512_fulldata_g demonstrate. As a common run the show, speedier printing speeds require higher spout temperatures, and bad habit versa . For high-quality comes about, it’s suggested to utilize spout temperatures between 205-210°C and print speeds of 50mm/s .
Layer tallness moreover plays a significant part in deciding print quality. For high-quality prints, layer statures between 60-100 microns are by and large considered ideal . Printing at layer statures underneath 60 microns can lead to altogether longer print times and potential distorting issues, indeed with PLA .
Post-processing Techniques
Post-processing methods can encourage upgrade the quality of yields produced by the places_512_fulldata_g demonstrate. In picture denoising assignments, for case, certain post-processing strategies have demonstrated successful in progressing comes about .
Blurring procedures such as middle channels, Gaussian obscure, and cruel channels have appeared to be especially successful in progressing denoising comes about . On the other hand, strategies like binarization, expansion, and disintegration are by and large not prescribed for making strides denoising results .
Interestingly, combining different post-processing strategies can lead to indeed superior comes about. In one case consider, combining four post-processing strategies progressed the competition score by 11%, from 0.26884 to 0.23933 . Be that as it may, it’s vital to note that expanding the number of post-processing strategies does not continuously ensure moved forward comes about .
Preparing Pictures for Inpainting
Effective planning of pictures is vital for accomplishing ideal comes about when utilizing the places_512_fulldata_g 目录 and its related places_512_fulldata_g.pth 路径. This prepare includes a few key steps and procedures to guarantee the best conceivable outcome.
Image Preprocessing Techniques
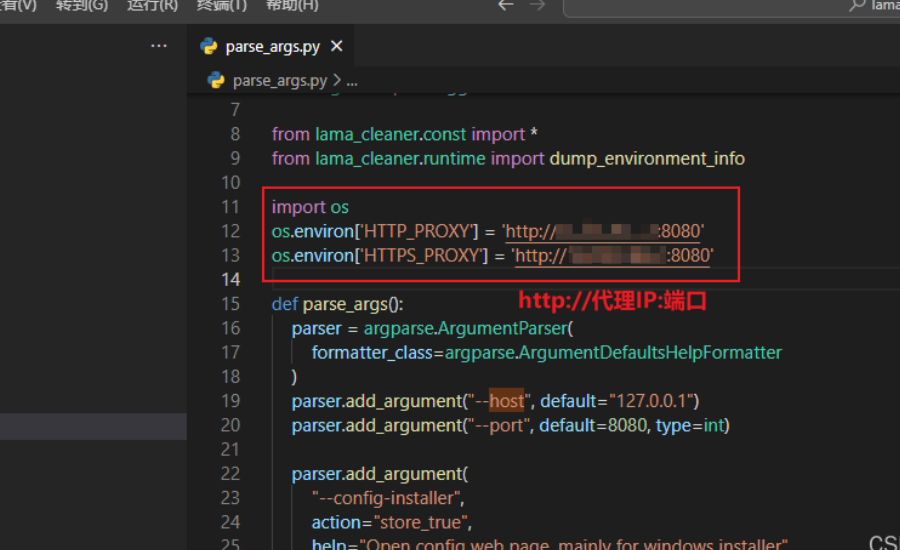
Image preprocessing is basic for controlling crude picture information into a usable arrange for inpainting errands. One of the essential methods is resizing pictures to a uniform measure, which is pivotal for machine learning calculations to work appropriately . For the places_512_fulldata_g 目录 demonstrate, pictures are regularly resized to 512×512 pixels amid preparing .
Normalization is another basic step, altering pixel concentrated values to a wanted extend, regularly between 0 and 1. This prepare can essentially make strides the execution of machine learning models . Also, differentiate improvement strategies such as histogram equalization can be connected to make strides the visual quality of pictures with destitute differentiate, possibly improving the execution of picture acknowledgment calculations .
Creating Viable Masks
Masking is a essential angle of inpainting with the places_512_fulldata_g 目录 demonstrate. To make covers, clients can utilize the draw apparatus in picture altering program or interfacing. The cover obscure slider permits for altering the accuracy of the mask’s edge, with higher values including more feathering . This feathering can offer assistance make more characteristic moves between the inpainted range and the unique image.
When working with nitty gritty ranges, such as fingers on a hand, it’s suggested to check the box for “inpainting at Full Resolution” . This choice zooms into the conceal range amid era, permitting for more exact and point by point inpainting results.
Handling Distinctive Picture Resolutions
The places_512_fulldata_g 目录 demonstrate illustrates noteworthy flexibility in taking care of different picture resolutions. In spite of being prepared on 512×512 pixel pictures, it can generalize shockingly well to much higher resolutions, indeed up to 2k . This capability permits clients to work with high-resolution pictures without noteworthy misfortune of quality or detail.
When managing with diverse resolutions, it’s critical to consider the adjust between preparing time and yield quality. Whereas the demonstrate can handle bigger pictures, handling time may increment with higher resolutions. Clients ought to explore with distinctive resolutions to discover the ideal adjust for their particular utilize case.
By carefully applying these preprocessing strategies, making successful covers, and understanding how to handle diverse picture resolutions, clients can maximize the potential of the places_512_fulldata_g show for their inpainting assignments.
Facts:
- places_512_fulldata_g Model:
- This is a specialized inpainting model designed for digital image restoration and enhancement.
- Built on the Stable Diffusion 1.5 architecture, it offers a dual-phase training process involving 595,000 steps of general training and 440,000 steps of inpainting-specific training.
- It is capable of restoring lost or damaged parts of an image with high accuracy by understanding both the image context and the missing areas.
- Key Features:
- The model uses a modified UNet architecture with additional input channels, which include one dedicated to the mask and four for encoding the hidden image.
- The inpainting model excels in both contextual understanding and edge consistency, filling missing areas with natural-looking extensions.
- Apart from inpainting, the model can also outpaint, extending images beyond their original boundaries.
- Preprocessing Requirements:
- Images need to be resized to 512×512 pixels for optimal performance.
- Normalization and contrast enhancement techniques are employed to improve model performance.
- Effective masking is essential, and using tools to adjust the mask edge feathering is recommended for smoother transitions.
- Optimizing Performance:
- Optimizing GPU usage through mixed-precision training and data preprocessing can enhance speed and efficiency.
- Balancing between speed and quality is crucial, especially when adjusting print settings for high-quality results.
- Post-processing methods like Gaussian blur can enhance image denoising.
- Resolution Flexibility:
- While trained on 512×512 images, the model works well with higher resolutions (up to 2k) without significant quality loss.
- Handling larger images increases processing time, so a balance between resolution and efficiency should be considered.
Summary:
The places_512_fulldata_g model is a powerful tool for digital image inpainting, specifically designed to restore missing or damaged parts of images with remarkable accuracy. Built upon the Stable Diffusion 1.5 architecture, this model uses a customized UNet design with extra input channels to handle both the original and masked areas of an image. Its dual-phase training process ensures detailed understanding of image context and missing regions.
To get the best results from this model, users should ensure proper image preprocessing, including resizing, normalization, and enhancing contrast. Masking is a critical part of the inpainting process, and fine-tuning the mask’s edges is essential for achieving seamless inpainting. The model also offers flexibility with resolutions, handling images up to 2k without significant loss of detail. Optimization strategies for GPU usage and a balance between speed and quality are key to ensuring efficient use of the tool in large-scale projects.
FAQs:
1. What is the places_512_fulldata_g model used for?
- The model is used for image inpainting, which means restoring missing or damaged parts of an image. It’s widely used in fields like photography, digital restoration, and creative arts.
2. How does the places_512_fulldata_g model work?
- It uses a modified UNet architecture combined with a dual-phase training process. This allows it to understand the full image context and fill in missing parts with high accuracy.
3. What are the key components of the places_512_fulldata_g model?
- Key components include input layers for the original image and mask, encoding layers for data processing, a UNet core for inpainting computations, and output layers for generating the final inpainted image.
4. Can the places_512_fulldata_g model handle high-resolution images?
- Yes, while it’s trained on 512×512 images, it can handle up to 2k resolution images with minimal loss of quality.
5. How should I prepare images for inpainting?
- Prepare images by resizing them to 512×512 pixels, normalizing the pixel values, and enhancing contrast. Additionally, create effective masks using editing tools and adjust the mask’s edges for smoother inpainting transitions.
6. What are the best practices for maximizing model performance?
- Optimize GPU usage with mixed-precision training, improve data handling for faster processing, and balance between speed and quality when performing inpainting. Post-processing techniques like Gaussian blur can help with noise reduction.
For more Information About Information visit idealrular.com